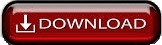
Our approach constrains 3D points to slide along their sensor view rays while neither adding nor removing any of them. We achieve this with 3D-VField: a novel method that plausibly deforms objects via vectors learned in an adversarial fashion.
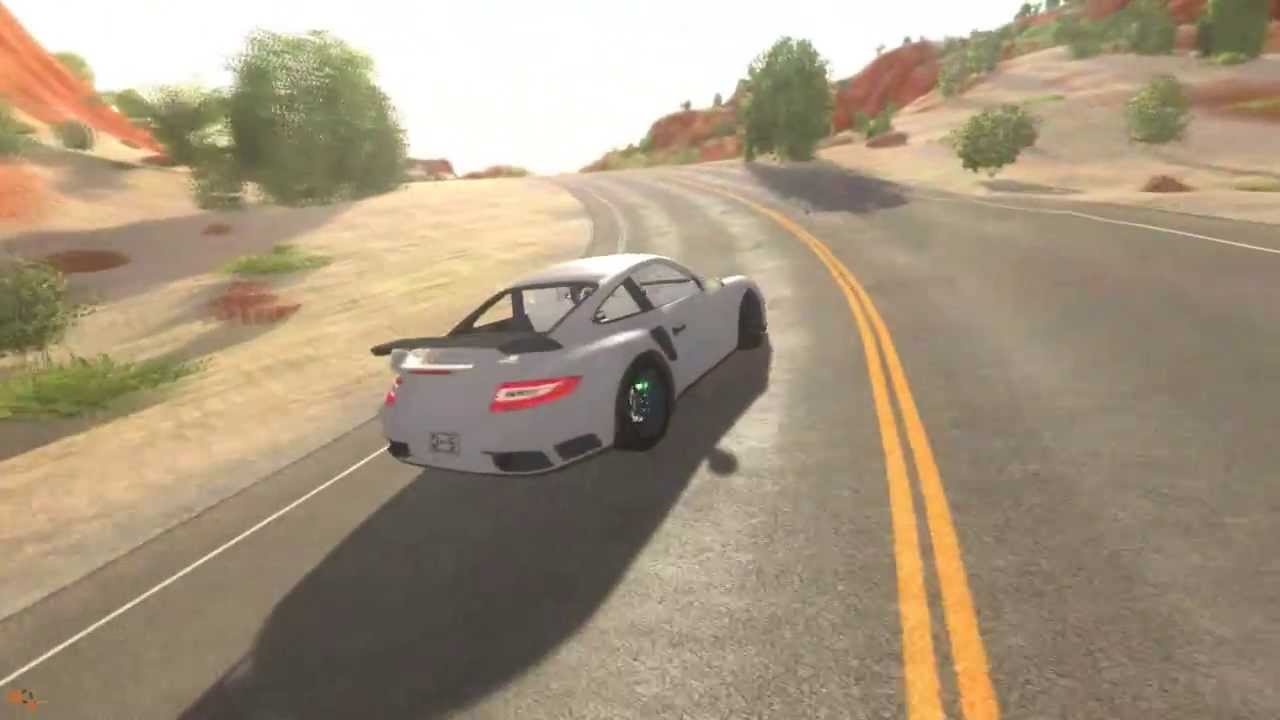
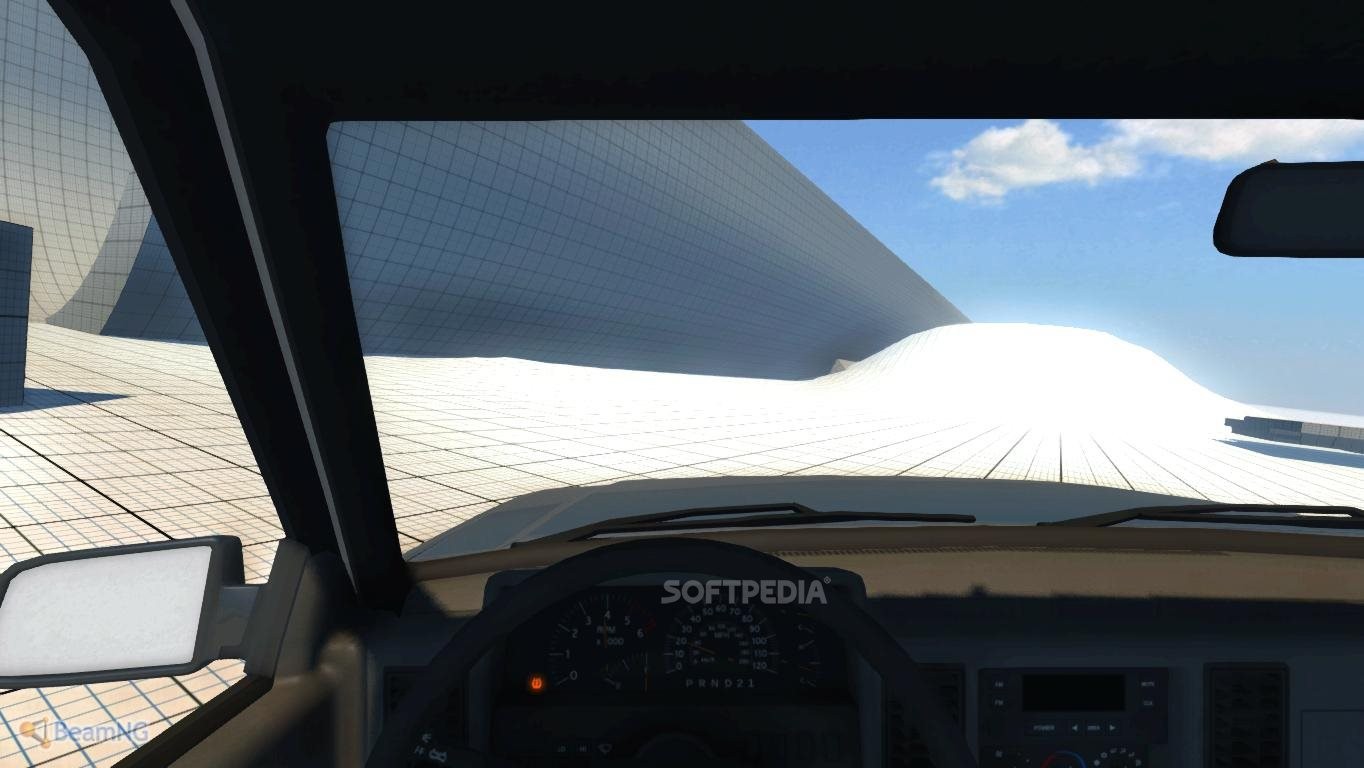
In this work, we substantially improve the generalization of 3D object detectors to out-of-domain data by taking into account deformed point clouds during training. As 3D object detection on point clouds relies on the geometrical relationships between the points, non-standard object shapes can hinder a method’s detection capability However, in safety-critical settings, robustness on out-ofdistribution and long-tail samples is fundamental to circumvent dangerous issues, such as the misdetection of damaged or rare cars.
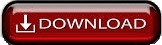